Cardiovascular disease (CVD) remains the primary cause of death in most industrialized and developing nations. The prevention of CVD depends on its timely diagnosis to initiation of cardioprotective therapies early on in the disease course. However, there remains a lack of an accurate risk model to predict an individual’s susceptibility to CVD.
A new Science Translational Medicine study describes an innovative proteomics-based model that predicts the risk of cardiovascular events within the next four years with higher accuracy than current clinical models.
Study: A Proteomic Surrogate for Cardiovascular Outcomes That Is Sensitive to Multiple Mechanisms of Change in Risk. Image Credit: alexacrib / Shutterstock.com
Introduction
The end-points for clinical trials of cardiovascular drugs include acute coronary events, hospitalizations, and deaths. However, this has led to some drugs going through advanced development before being found to increase cardiovascular risk. Comparatively, other drugs with promising cardioprotective effects have not been approved for such indications because these effects were shown too late in the development process.
The traditional cardiovascular risk factors are also not particularly useful in predicting risk in people with known CVD but controlled levels of cholesterol and blood pressure, those with multiple chronic illnesses, and the elderly.
Finally, many of these risk factors including age, sex, a history of diabetes and certain imaging factors do not change to reduce the calculated effect of risk reduction when using agents that act independently of these factors. Thus, the researchers of the current study sought to generate and test a new model of cardiovascular risk that would use newer biomarkers, as an outcome measure instead of the earlier clinical endpoints.
“The idealized requirements of precise, sensitive prognostics that respond agnostically and reliably to all changes in outcomes regardless of intervention mechanism are key features of a surrogate end point.”
Herein, the researchers created a proteomics-based prognostic score that would be predictive of actual cardiovascular outcomes within a relatively short time frame, while also including all known mechanisms and allowing the model to be responsive to changes in the outcome. If successful, this score would be useful for Phase II studies for drugs used in the prevention and treatment of CVD and diabetes, as well as an endpoint for the accelerated approval of breakthrough drugs.
Finally, the researchers also anticipate that their score could be used to allocate drugs selectively to individuals at risk of CVD and measure patient outcomes.
Study findings
The researchers measured 5,000 proteins in each sample of plasma and applied machine learning to the results to develop a prognostic model. The model used 27 proteins and predicted the absolute risk that any of the multiple components that made up the composite endpoint, some of which included heart attack, stroke, hospitalization for heart failure, and mortality from any cause, would occur within the next four years.
This was tested on multiple cohorts with several comorbidities and changes in the parameters were measured over time. Overall, over 11,600 participants with a four-year outcome were included in the study.
At this point, 22% of the population had experienced one or more of these events for an event number of 2,500. These events consisted of 622 hospitalizations for cardiac failure, 601 heart attacks, and 345 strokes.
Of the proteins used in this model, 14 showed a positive correlation, and 13 a negative correlation. These proteins correspond to ten or more biological processes, such as those involved in maintaining blood volume and sodium excretion, the formation of vesicles, angiogenesis, and the glomerular filtration rate.
Mendelian analysis was used to explore possible cause-and-effect relationships between 16 of these proteins, which were found in the available PheWAS database. This showed that a dozen of them were linked to one or more traits related to CVD.
The current model could also predict event rates over a wide range of values. The highest and lowest quintiles of predicted risk showed a five- to seven-fold increase in the event rates at four years in the first two validation datasets. The metacohort, which included all 11,600 participants, also showed a seven-fold increase in the four-year event rate.
The scientists also created four risk categories based on the protein values. These had four-year event rates of 6%, 11%, 20%, and 43%, respectively, in the six studies that made up the meta-cohort. This corresponded to low, low-medium, medium-high, and high risk, respectively. Moreover, the median lag to the event was less than two years overall as compared to 1.5 years in the highest quintile.
The model also responded in the right direction to adverse and beneficial changes in the protein-predicted risk. For instance, in the ACCORD trial, part of the dataset used here, the risk of CVD increased by 6% over two years, correctly foreseeing a future adverse event after the second sample was taken. The PRADA trial also reflected a 6% increase in risk from the baseline within three months of initiating anthracycline chemotherapy.
Beneficial changes were seen in response to the glucagon-like peptide 1 (GLP-1) receptor agonist exenatide in the EXSCEL trial. The absolute four-year event risk was reduced by 0.8% in just one year as compared to the predicted reduction of 1.5% with this model. In the DiRECT trial, again, almost 50% remission of diabetes was achieved in one year, where the absolute risk was predicted to be reduced by 6.7% when compared to the standard diet group.
Finally, the model predicted no effect of treatment correctly in the subset of the ACCORD trial that had intensive diabetic control, and for patients in the PRADA trial in response to either beta-blockers or angiotensin receptor blockers.
The model also predicted higher risks with a variety of conditions that are known to increase the incidence of event rates, such as breast cancer treatment, those with prior events, and those currently smoking/diabetic/with a history of cancer. In the first case, in the PRADA study, the predicted risk was 14% higher as compared to the earlier prediction of 5% from another cohort of matched women.
Implications
The model developed in the current study showed a consistent correlation between the event rate and predicted absolute risk, which surpasses currently available prognostic models. Moreover, the current model had more than double the dynamic range and reclassified cardiovascular risk better. Moreover, this model is biologically coherent, as the various biological processes that are involved in cardiovascular health are mediated and regulated by proteins.
“The reliable identification of individuals with an observed event rate of >50% and a median time to event of 18 months is of clinical and economic relevance.”
Proteins also change with environmental conditions, depending on the level of gene expression. All 27 proteins used in the model were associated with processes that predict a higher cardiovascular risk. Of these, 16 and 12, respectively, were part of a database exploring the correlation between these proteins and the genome, and were connected causally with one genetic factor for CVD or one of its risk factors.
Under conditions of positive, negative, and neutral changes in the risk factors, this protein-based model showed true reductions, increases, or no change in the predicted absolute risk. When other conditions associated with an increase in cardiovascular events were incorporated to the analysis, including smoking and diabetes, the model continued to predict elevated risk correctly. It also predicted that untreated high systolic blood pressure and high lipid levels in the same group would enhance the risk.
This shows that the surrogate is universal and will respond to a change in outcome, irrespective of the mechanism. This multi-protein model is also more sensitive to risk factors than individual biomarkers.
Further work along the same lines may provide a very necessary universal surrogate endpoint for cardiovascular risk.
- Williams, S. A., Ostroff, R., Hinterberg, M. A., et al. (2022). A Proteomic Surrogate for Cardiovascular Outcomes That Is Sensitive to Multiple Mechanisms of Change in Risk. Science Translational Medicine. doi:10.1126/scitranslmed.abj9625.
Posted in: Device / Technology News | Medical Research News | Medical Condition News
Tags: Angiogenesis, Angiotensin, Anthracycline, Blood, Blood Pressure, Breast Cancer, Cancer, Cancer Treatment, Cardiology, Cardiovascular Disease, Chemotherapy, Cholesterol, Chronic, Diabetes, Diet, Drugs, Exenatide, Gene, Gene Expression, Genetic, Genome, GLP-1, Glucagon, Heart, Heart Attack, Heart Failure, Imaging, Machine Learning, Medicine, Mortality, Protein, Proteomics, Receptor, Smoking, Stroke
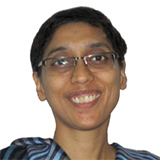
Written by
Dr. Liji Thomas
Dr. Liji Thomas is an OB-GYN, who graduated from the Government Medical College, University of Calicut, Kerala, in 2001. Liji practiced as a full-time consultant in obstetrics/gynecology in a private hospital for a few years following her graduation. She has counseled hundreds of patients facing issues from pregnancy-related problems and infertility, and has been in charge of over 2,000 deliveries, striving always to achieve a normal delivery rather than operative.
Source: Read Full Article