Basic components of network medicine
Predicting causative genes of human disease
Application of network medicine to disease classification and identification
Network pharmacology: applying networks to disease treatment and drug design
The future outlook of network medicine
References
Further reading
The human interactome represents a vastly complex and daunting mesh of communication networks across cells and organs. The number of protein-coding genes stretches to around 25,000, and the number of functionally critical relationships is expected to surpass 100,000.

Image Credit: optimarc/Shutterstock.com
Out of this complexity arises a key hypothesis that the outcomes and phenotypes of disease are seldom the product of a single genetic abnormality and are more likely to result from a complex network of relationships in the interactome.
Network medicine can systematically enhance the ability to address human disease through the identification of disease pathways, causative genetic factors, and molecular phenotypic relationships that previously appeared distinct. This interactome-wide approach is a necessary tool in the identification of novel disease-causing mutations, biomarkers, and drug targets.
Basic components of network medicine
Modules represent clusters of components, or nodes, within a network region showing high levels of linkage and connectivity. These components are often the subject of disease gene identification and drug design, given their significance to disease-related phenotypes. These modules can often comprise small groupings of highly interacting nodes, called hubs, which tend to be controlled by essential genes.
Groups of interconnected nodes can form subnetworks within an overall network, referred to as a motif. These collections have often been implicated in biological functions like negative feedback loops and oscillators.
Predicting causative genes of human disease
Genome-wide association (GWA) studies represent the conventional approach to identifying causal genes of a disease, producing a range of candidates in need of follow-up. However, elucidating the true genetic culprit is still a difficult and involved process needing improvement. Network-based approaches can advance this predictive ability.
Linkage-based methods to causative gene identification operate under the assumption that disease phenotypes will be associated with the disease protein and its interactors. Implementing this into network modeling can lead to 1,000-fold enrichment over the random selection and has directly informed the association of Janus kinase 3 (JAK3) with severe combined immunodeficiency syndrome.
Disease module-based methods focus on identifying and investigating the network module of a certain disease. Modules and subnetworks are identified in constructed interactomes, and all nodes within a disease module are treated as potential causal elements. These approaches have been applied to the study of cancer, Huntington’s disease, schizophrenia, and type two diabetes.
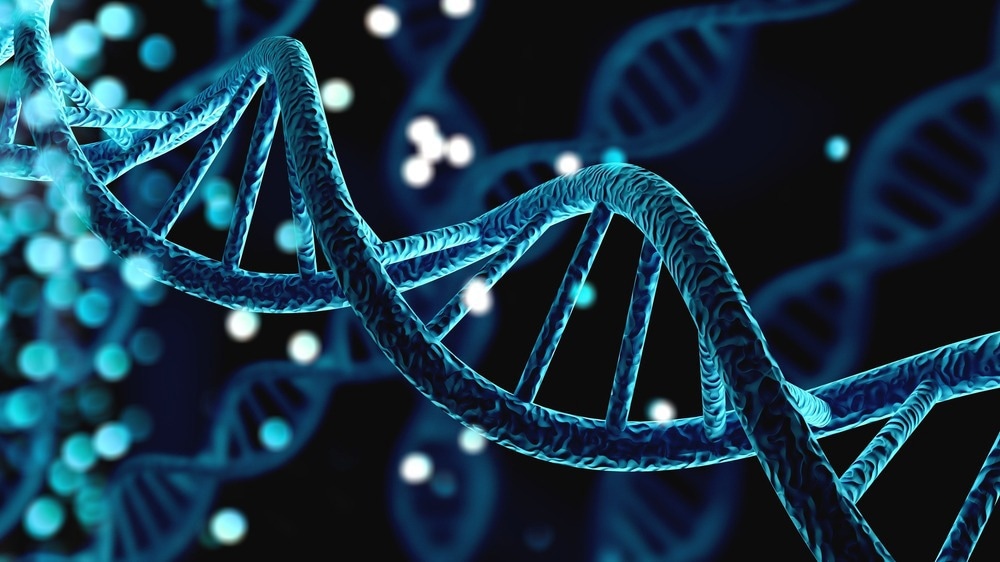
Image Credit: Billion Photos/Shutterstock.com
Diffusion-based methods use known disease genes to investigate closely connected and related pathways using algorithms dispatching ‘random walkers’ from a disease gene throughout the interactome. The frequency of random walkers at a certain node indicates an increased probability of a relationship between the nodes and the disease gene. These methods have currently been applied in the study of Alzheimer’s disease.
Application of network medicine to disease classification and identification
The classification of human diseases has traditionally relied on a combination of prior knowledge of clinical manifestations and correlations with clinical observations. This approach has been deemed insufficient in many respects, including its limited ability to distinguish resolutely between conditions and the inability to diagnose those at the preclinical stage reliably.
The incorporation of network medicine into this area of pathobiology allows diseases to be classified not as a single manifestation or mutation but as a result of interconnected networks with multiple significant nodes. This approach accounts for the potential effects of environmental influences on disease, such as post-translational modifications and epigenetic factors.
An example of the benefits of this approach would be in the examination of the hemoglobin β-chain mutation that causes sickle cell anemia. In the context of a network, this gene acts as a node with a wide array of interactions with other genes responsible for the modulation of disease phenotypes. In some cases, these interactors cause intermediate phenotypes that may complicate conventional classification approaches.
Network pharmacology: applying networks to disease treatment and drug design
Due to the aforementioned complexity of human diseases and drug mechanisms of action, the application of network medicine to drug discovery and design has the potential to inform investigative efforts and tailor drugs to specific diseases.
The search for candidate drugs can be labor-intensive and daunting, involving screening and analyzing massive compound collections and libraries. Networks establish that dysfunction caused by disease, on a cellular level, is confined to network nodes. Therefore, these networks can help center research efforts on more promising candidates by focusing on those compounds that affect node activity.
Additionally, the complex nature of disease often means that dug candidates with promising activity against their target in vitro can lose effectivity once studied in vivo. This failure in activity is an example of the reductionist nature of the one disease-one target theory.
Confounding factors like multiple interactors or binding partners with cascading effects may affect activity in ways that networks may better predict. For example, metabolic networks have been employed to characterize drug-induced enzymatic activity in studying antibacterial agents.
The future outlook of network medicine
Network medicine allows for more nuanced, systems-level approaches to the identification, prevention, and treatment of disease. The precision and wide applicability offered by these approaches hold a great deal of promise for the future of combatting complex human diseases.
References
- Barabási, A.L., Gulbahce, N., Loscalzo, J. (2011). Network medicine: a network-based approach to human disease. Nature Reviews Genetics, 1(12), pp.56-68. doi: 10.1038/nrg2918
- Lee, L.Y.H., Loscalzo, J. (2019). Network Medicine in Pathobiology. The American Journal of Pathology, 7(189), pp.1311-1326. doi: 10.1016/j.ajpath.2019.03.009
- Morselli Gysi, D., et al. (2021). Network medicine framework for identifying drug-repurposing opportunities for COVID-19. Proceedings of the National Academy of Sciences, 19(118), e2025581118, doi: 10.1073/pnas.2025581118
Further Reading
- All Medicine Content
- Herbal versus Synthetic Medicines
- The Relationship Between Climate Change and Global Social Medicine
- The Replication Crisis in Biomedicine
- What is Global Social Medicine?
Last Updated: Apr 12, 2023
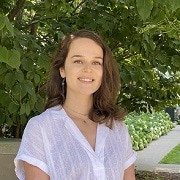
Written by
Antonia Du Bois
Antonia was not always a scientist, beginning her higher education with a joint science and arts degree at the University of Guelph in 2015. After exploring her interests in a range of non-scientific areas including studio art, theatre studies, and classics, Antonia changed course in 2016 to pursue a Bachelor of Science in Molecular Biology and Genetics.
Source: Read Full Article